By Claire Wright | April 11, 2022
In the previous blog, Origins of Repeat Photography as Tool for Monitoring Landscape Change, I argued that repeat photography is an effective and flexible technique for examining change in mountain landscapes. With historic photographs taken as early as the mid-nineteenth century, the images provide far greater temporal depth than satellite or aerial imagery. The original images often have a high spatial resolution and may provide better coverage of steep slopes compared to orthographic views. Despite these benefits, researchers using repeat photography are only just starting to delve into quantitative spatial analysis, an area where other remote sensing techniques excel. Researchers at the Mountain Legacy Project (MLP) are at the forefront of innovation in the development of oblique image analysis tools and workflows. However, much work remains to streamline the analysis process and improve the robustness of the methodologies involved.
The majority of repeat photography studies have relied on qualitative comparisons of the conditions in historical and modern images. It was not until the early 2000s that some researchers began to make forays into quantitative analysis. Jeanine Rhemtulla and her colleagues, in the early days of what is now the MLP, combined qualitative and quantitative methods to assess change in repeated oblique images of the Canadian Rockies. They overlayed 20 manually classified image pairs on a grid then used a transition matrix to track relative vegetation change at the pixel-level between each pair. However, as the authors acknowledge, their results are biased since they rely on the assumption that pixel units remained constant within and between photographs which is not the case. At the same time, researchers at Colorado State University developed a Geographic Information System (GIS) workflow involving image-to-image rectification, image sub-setting, and automated classification to analyze landscape changes in the Rocky Mountains in Colorado. While the use of image sub-setting and pairwise comparisons reduced bias created by differences in scale between pixels, significant error was still present. Subsequent developments involved labour-intensive grid-overlay techniques in various GIS. These early studies represented the first inroads into quantifying landscape change through repeated oblique imagery. They were limited to relative comparisons and there was little evaluation of accuracy.
“Considering the aforementioned challenges associated with quantifying changes visible in terrestrial oblique angle images, no one has yet used historic photographs to conduct a landscape-level (100’s to 1000’s km2) quantitative assessment of vegetation pattern change across a century”
– Stockdale et al. (2015)
Quantitative spatial analysis of repeat photography continued to advance with computer-based methods for spatially referencing oblique images. Digital monoplotting, the photogrammetric practice of relating a single unrectified aerial or oblique image to a digital elevation model (DEM), was pioneered in the early 1970s. However, it took significant advancements in computing power, DEM quality, and GIS before the technique began to develop in earnest. Early monoplotting systems include two FORTRAN programs (CAM-LOC/OP-XFOR), an integrated workflow of three separate programs for monoplotting applications in forestry, the JUKE method, and a georeferencing algorithm paired with viewshed analysis in ArcGIS Info. None of these tools were widely adopted due to being task-specific, inaccurate, and computationally demanding. Then, researchers out of the Swiss Federal Institute for Forest, Snow and Landscape Research developed a new monoplotting system, the WSL Monoplotting Tool. Unlike previous attempts, the tool was created with the goal of providing a user-friendly interface and end-products to be integrated with conventional GIS. As well, a workflow was subsequently developed to extract raster data from the images using the Viewshed tool in ArcGIS Desktop and parameters derived from the WLS Monoplotting Tool. The tool has been used in a number of land cover change studies since its release. Research indicates that it can result in geographic products with high spatial accuracy paired with high resolution elevation data and careful control point placement. However, there are still limitations on data handling and cross-talk between software programs. Researchers at MLP are currently working on an in-house, web-based monoplotting tool with the possibility of integrating it with a commonly used GIS such as QGIS which has built-in viewshed creation tools. The development of effective monoplotting tools for oblique imagery represent a vital step in the advancement of quantitative analysis methods for studying landscape change in repeat photography.
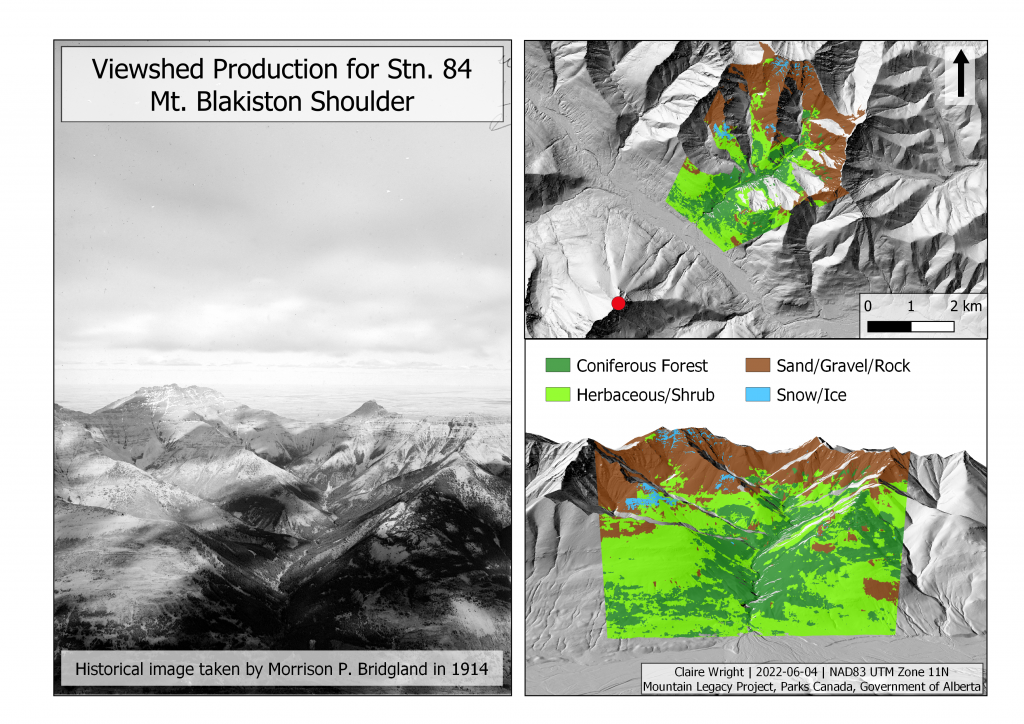
Another important element of landscape change analysis using repeat photography is landcover classification. Most studies involve manual classification using various software programs such as Photoshop, ArcGIS, and Image Labeller. However, these methods are time-consuming and labour-intensive. This is in contrast to aerial and satellite imagery where a plethora of automated classification systems exist. In 2015, a machine-learning segmentation algorithm was created to fill this gap for classifying images from the MLP collection. The method produces binary maps segmented into forested and non-forested classifications. Separately, Buscombe and Richie (2018) who used a deep-learning architecture for segmenting landscape classes from multiple image sources, including oblique photography. However, their study still handled vegetation as a single class. Bayr and Puschmann (2019) proposed one of the first automated classification of oblique images using multiple classes for vegetation (woody and herbaceous). Finally, Spencer Rose, a researcher with MLP, recently developed a Python tool that uses a deep learning semantic segmentation algorithm for the classification of land cover in oblique photographs. The classifier, PyLC, uses deep convolutional neural networks implemented with U-net and DeepLab V3+ segmentation models. The package uses a novel threshold-based data augmentation algorithm in image pre-processing as well as a conditional random fields model to improve segmentation accuracy. Rose’s work, developed using the MLP collection, offers a path forward for automating and streamlining quantitative analysis of landcover change using repeat photography.
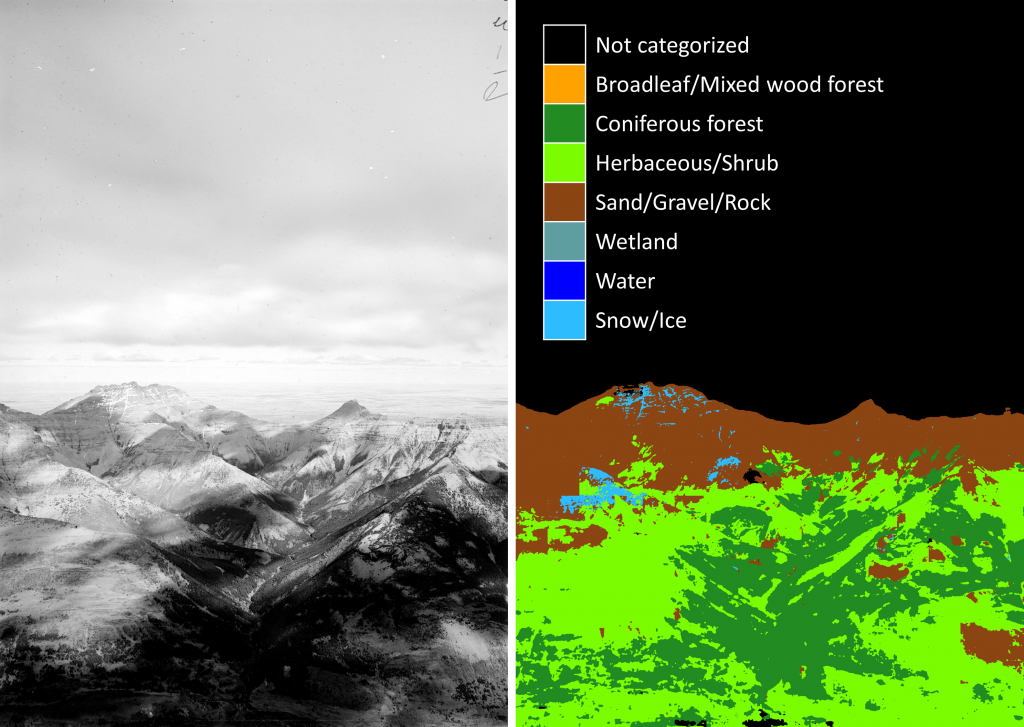
Despite major advances in quantitative analysis methods for repeat photography, the various programs and software platforms remain fairly isolated. This is partially solved by the Image Analysis Toolkit (IAT), a suite of online tools developed for working with MLP images. IAT hosts several tools for the alignment and visual comparison of paired photographs. It also allows the user to manually classify landcover types in the images using polygons and other drawing tools. Recently, a monoplotting process was added allowing the production of georeferenced viewsheds of classified. IAT has been used in a range of research including an analysis of human development and vegetation change in the Bow River watershed, Alberta, Canada, an assessment of alpine treeline ecotone shift in the West Castle Watershed (also in Alberta), and a recent comprehensive study of ecological change in the Canadian Rocky Mountains. However, some technical challenges remain such as the need to use external software to re-size the images and the lack of accuracy assessment. Research is ongoing into the integration of an automated classification algorithm into the toolset. The future of quantitative analysis in repeat photography lies in the development and refinement of end-to-end workflows such as the one available through IAT. There is also potential to develop an integrated or separate workflow in a mainstream GIS such as QGIS or ArcGIS which would greatly enhance the spatial analysis possibilities.
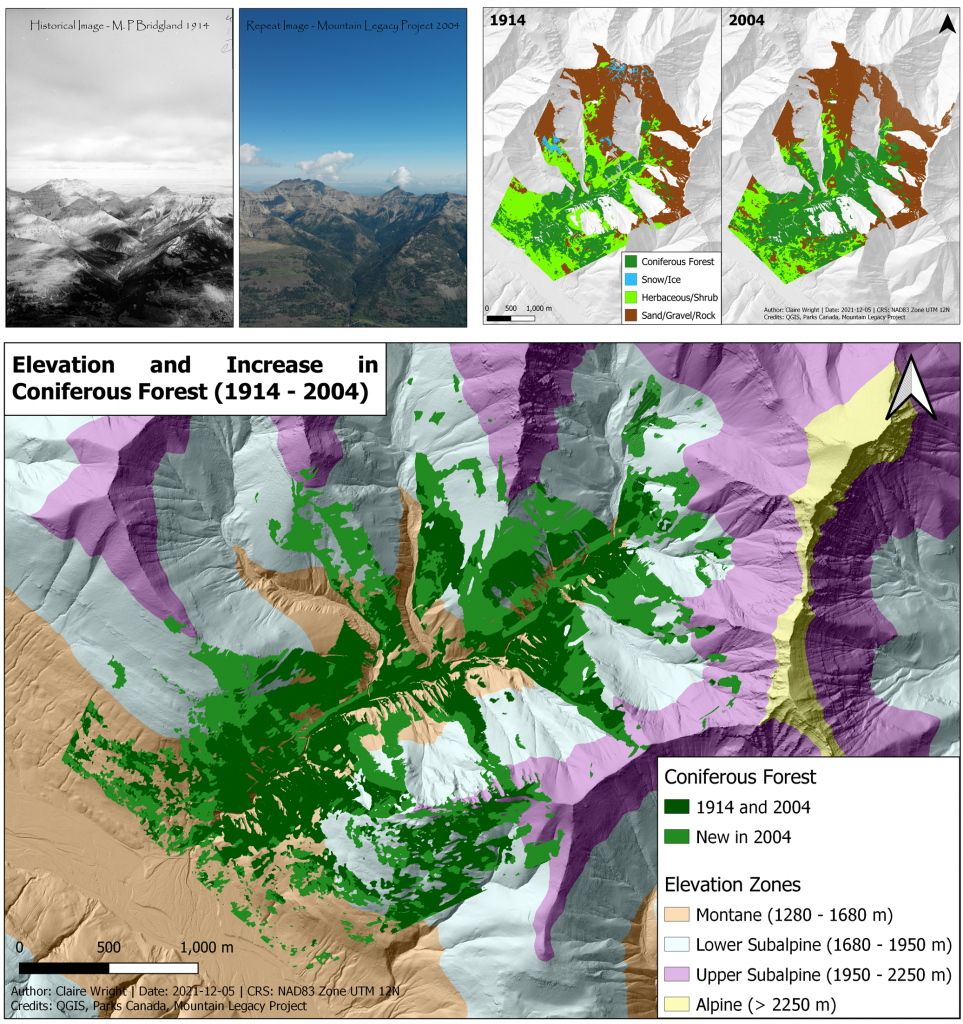
The temporal depth, extensive coverage, and high spatial resolution of oblique images make repeat photography an ideal tool for studying change in mountain environments. Advances are now being made in the quantitative spatial analysis of oblique images, an area where repeat photography lags behind other remote sensing techniques. Recent progress in terms of image preparation, monoplotting, and automated image classification is rapidly bridging the gap. The current challenge lies in the development of user-friendly, repeatable workflows from image selection to the actual analysis of spatial products. The Image Analysis Toolkit developed through the Mountain Legacy Project offers one promising path forward. Future research should focus on end-to-end workflows that incorporate automated classification, provide robust accuracy assessment, and integrate with conventional spatial analysis platforms.
References
[1] Kull, C. A. (2005). Historical landscape repeat photography as a tool for land use change research. Norsk Geografisk Tidsskrift, 59(4), 253–268.
[2] Pickard, J. (2002). Assessing vegetation change over a century using repeat photography. Australian Journal of Botany, 50(4), 409–414.
[3] Sanseverino, M. E., Whitney, M. J., & Higgs, E. S. (2016). Exploring landscape change in mountain environments with the Mountain Legacy Online Image Analysis Toolkit. Mountain Research and Development, 36(4), 407–416.
[4] Stockdale, C. A., Bozzini, C., Macdonald, S. E., & Higgs, E. (2015). Extracting ecological information from oblique angle terrestrial landscape photographs: Performance evaluation of the WSL Monoplotting Tool. Applied Geography, 63, 315–325.
[5] Rogers, G. F. (1982). Bibliography of repeat photography for evaluating landscape change. University of Utah Press.
[6] Rhemtulla, J. M., Hall, R. J., Higgs, E. S., & Macdonald, S. E. (2002). Eighty years of change: Vegetation in the montane ecoregion of Jasper National Park, Alberta, Canada. Canadian Journal of Forest Research, 32(11), 2010–2021.
[7] Manier, D. J. & Laven, R. D. (2002). Changes in landscape patterns associated with the persistence of aspen (Populus tremuloides Michx.) on the western slope of the Rocky Mountains, Colorado. Forest Ecology and Management, 167, 263–284.
[8] Roush, W., Munroe, J. S., & Fagre, D. B. (2007). Development of a spatial analysis method using ground-based repeat photography to detect changes in the alpine treeline ecotone, Glacier National Park, Montana, U.S.A. Arctic, Antarctic, and Alpine Research, 39(2), 297–308.
[9] Watt-Gremm, G. D. (2007). Taking a good long look: disturbance, succession, landscape change and repeat photography in the upper Blakiston Valley, Waterton Lakes National Park [Doctoral dissertation].
[10] Makarovič, B. (1973). Digital monoplotters. The ITC journal, 1973(4), pp. 583–600.
[11] Bozzini, C., Conedera, M., & Krebs, P. (2012). A new monoplotting tool to extract georeferenced vector data and orthorectified raster data from oblique non-metric photographs. International Journal of Heritage in the Digital Era, 1(3), 499–518.
[12] Doytsher, Y., & Hall, J. K. (1995). FORTRAN programs for coordinate resection using an oblique photograph and high-resolution DTM. Computers and Geosciences, 21(7), 895–905.
[13] Aumann, G., & Eder, K. (1996). An integrated system of digital monoplotting and DTM modelling for forestry applications. International Archives of Photogrammetry and Remote Sensing, 31, 221–225.
[14] Aschenwald, J., Leichter, K., Tasser, E., & Tappeiner, U. (2001). Spatio-temporal landscape analysis in mountainous terrain by means of small format photography: A methodological approach. IEEE Transactions on Geoscience and Remote Sensing, 39(4), 885–893.
[15] Corripio, J. G. (2004). Snow surface albedo estimation using terrestrial photography. International journal of remote sensing, 25(24), 5705-5729.
[16] Gabellieri, N., & Watkins, C. (2019). Measuring long-term landscape change using historical photographs and the WSL Monoplotting Tool. Landscape History, 40 (1), 93-109.
[17] McCaffery, D., & Hopkins, C. (2020). Repeat oblique photography shows terrain and fire-exposure controls on century-scale canopy cover change in the alpine treeline ecotone. Remote Sensing, 12(10).
[18] Stockdale, C. A., Macdonald, S. E., & Higgs, E. S. (2019). Forest closure and encroachment at the grassland interface: A century-scale analysis using oblique repeat photography. Ecosphere, 10(1).
[19] Bayr, U., & Puschmann, O. (2019). Automatic detection of woody vegetation in repeat landscape photographs using a convolutional neural network. Ecological Informatics, 50, 220-233.
[20] Fortin, J. (2015). Lanscape and biodiversity change in the Willmore Wilderness Park through repeat photography [Master’s thesis]. University of Victoria.
[21] Taggart-Hodge, T. (2016). A century of landscape-level changes in the bow watershed, Alberta, Canada, and implications for flood management [Master’s thesis]. University of Victoria.
[22] Rose, S. (2020). An evaluation of deep learning semantic segmentation for land cover classification of oblique ground-based photography [Master’s thesis]. University of Victoria.
[23] Jean, F., Albu, A. B., Capson, D., Higgs, E. S., Fisher, J. T., & Starzomski, B. M. (2015). The mountain habitats segmentation and change detection dataset. Proceedings – 2015 IEEE Winter Conference on Applications of Computer Vision, WACV 2015, 603-609.
[24] Buscombe, D., & Ritchie, A. (2018). Landscape classification with deep neural networks. Geosciences, 8(7), 244-267.
[25] Higgs, E. S., Sanseverino, M., Whitney. M., & Fortin, J. (2020). Advances in visual applications: visualizing & quantifying landscape change in SW Alberta using Mountain Legacy Project photography.fRI Research Landscapes in Motion.
[26] Trant, A., Higgs, E., & Starzomski, B. M. (2020). A century of high elevation ecosystem change in the Canadian Rocky Mountains. Scientific Reports, 10(1).
[27] Veblen, T. T., & D. C. Lorenz. (1991). The Colorado front range: A century of ecological change. University of Utah Press.
[28] Skovlin, J. M., & Thomas, J. W. (1995). Interpreting long-term trends in blue mountain ecosystems from repeat photography ( No. 315). USDA, Pacific Northwest Research Station.